Investigating the interplay between optimizer and architecture in Deep Learning, and new networks for long-range reasoning.
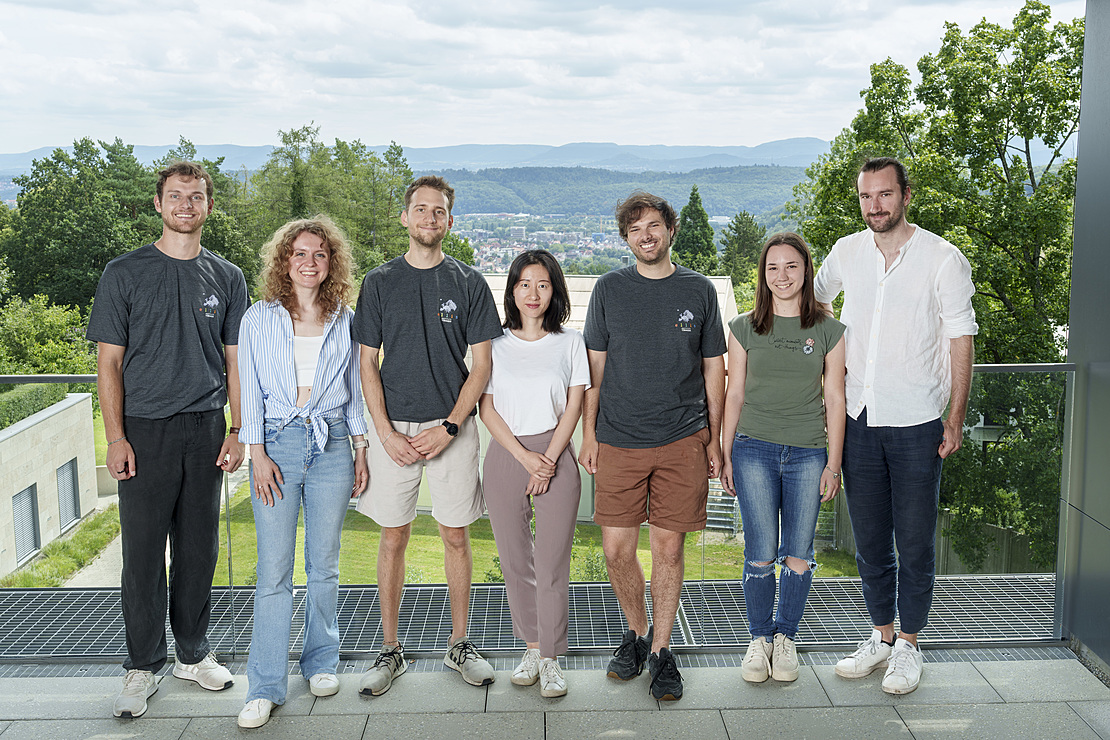
The purpose of our research is to design new optimizers and neural networks to accelerate technology and scientific discovery with Deep Learning. Our approach is theoretical, with a strong focus on optimization theory as a tool for understanding the challenging dynamics of modern neural networks.
We strongly believe deep learning will revolutionize science and technology, offering solutions to society's most pressing challenges. With a stronger theoretical foundation, we envision a future where scientists and engineers, regardless of their resource limitations, can leverage powerful and reliable deep learning solutions to help make the world a better place.
If you like our mission, please apply for CLS, ELLIS, IMPRS-IS PhD Programs (deadline Nov 15th).
Teaching at the University of Tübingen: Nonconvex Optimization for Deep Learning (Winter Semester 24/25), Details here.