Investigating the feasibility of technical solutions to safety and efficiency in machine learning.
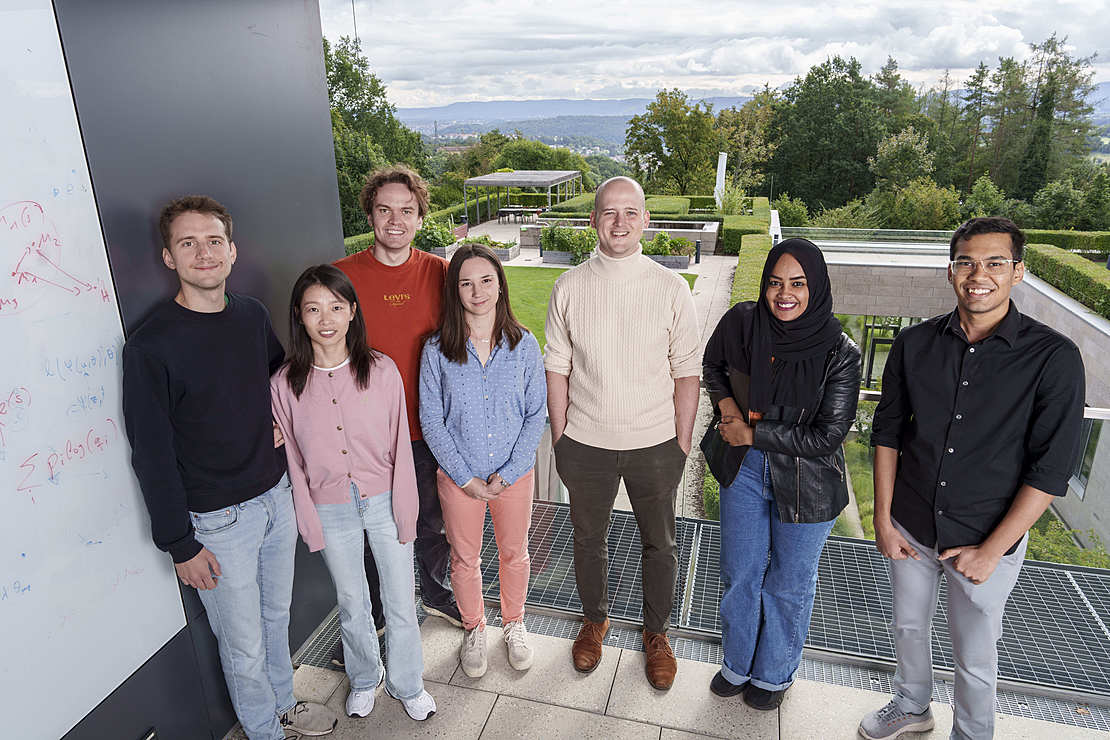
The SEAL group is interested in questions of safety and efficiency in modern machine learning. There are a number of fundamental machine learning questions that come up in these topics that we still do not understand well. In safety, examples are questions about the principles of data poisoning, the subtleties of water-marking for generative models, privacy questions in federated learning, or adversarial attacks against large language models. Can we ever make these models “safe”, and how do we define this? Are there feasible technical solutions that reduce harm? Further, the research group is interested in questions about the efficiency of modern AI systems, especially for large language models. How efficient can we make these systems, can we train strong models with little compute? Can we extend the capabilities of language models with recursive computation? How do efficiency modifications impact the safety of these models?